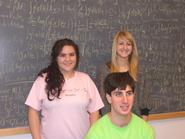
Sitting in front of a computer screen, scientists spend hours staring at satellite images of outer space, searching for exploding supernovae. But surprisingly, visual identification is the main way that astronomical laboratories identify supernovae. Led by Assistant Professor of Physics Natalia Connolly, Ileana Becerra ’11, Anne Vilsoet ’11 and Will Eagan ’11 are creating a smarter computer program that will more reliably detect supernovae in satellite images.
Supernovae are the fireworks that are emitted after a star dies. When a dead star (a white dwarf) becomes too dense, it can explode, emitting so much radiation that it even outshines a galaxy. Although supernovae vary depending on the reason behind their explosion, the team is focusing on the brightest type, type 1a supernovae. The explosion occurs when a white dwarf (a small star with incredible mass and small volume) collects so much matter from its fellow binary star, which is one step behind in its stellar life cycle and is still a red giant (an enlarged star with high volume and comparatively low mass). Since all type 1a supernovae emit the same amount of radiation, they are known as standard candles, luminous objects in relation to which scientists can calculate astronomical distances.
This summer, the team is facing the epic digital-age battle of humans versus computers—except they are rooting for the computer, trying to make it more efficient than themselves. They compose synthesized images of outer space based on a program that randomly assigns a value to each pixel. To know the range of values for each pixel, the program draws from real satellite image data. Once the images are simulated, the team tries to pick out the significant supernovae from the “noise,” or the confusing jumble of radiation in the background. They then run the image through a computer program that Brian Connolly created and compare the number of supernovae the computer found to the number they found, hoping that the computer found more.
But more data is about to arrive, and in rapid succession. The SNAP (Supernova Acceleration Probe) satellite, a joint venture of NASA and the U.S. Department of Energy, would take even more resolved pictures of the night sky—and being able to rapidly and effectively distinguish supernovae is necessary for the processing of the data that SNAP collects. Although it is still only a proposed mission, SNAP could launch as early as 2020, so the race for effective supernova-processing software is on.
Today, algorithmic software to detect supernovae exists, but it is still inaccurate and inefficient. The team’s software takes about a day to run a single image—a relatively short amount of time. But, if they can program the software to process the images even faster while maintaining accuracy, it can be much more marketable. And if they continue to have the success they have already found in reprocessing and reanalyzing the algorithms, the team may be achieve its goal of making a more accurate program.
Besides the many hours she spends coding, Becerra enjoys doing “anything musical”; she plays piano, guitar and drums, and has performed as a professional Latin singer in her hometown of Venice, Calif. On campus she sings opera and is a member of the sorority AXL.
Vilsoet spent last semester in the Hamilton program in Paris, where she had plenty of opportunities to pursue her hobbies of going to museums and traveling. Her upcoming senior thesis in physics will most likely pertain to the subject of supernova detection, although she will focus more on the statistical analysis than computation and programming.
Eagan is a member of the cycling team and the president of the Republican club. This summer, he is also simultaneously working on another research project with Professor Connolly comparing algorithms for statistical modeling that will help determine how to create the “best model” for any given situation. As a model becomes more complex, it also becomes more accurate. But to avoid over-complication, different algorithms introduce a penalty for increasing the complexity. The “best model” is one that comes closest to achieving equilibrium between complexity factor and the penalty.
Becerra graduated from New Roads High School in Santa Monica, Calif., Vilsoet from Edgemont High School in Scarsdale, N.Y., and Eagan from Hingham High School in Hingham, Mass.
Supernovae are the fireworks that are emitted after a star dies. When a dead star (a white dwarf) becomes too dense, it can explode, emitting so much radiation that it even outshines a galaxy. Although supernovae vary depending on the reason behind their explosion, the team is focusing on the brightest type, type 1a supernovae. The explosion occurs when a white dwarf (a small star with incredible mass and small volume) collects so much matter from its fellow binary star, which is one step behind in its stellar life cycle and is still a red giant (an enlarged star with high volume and comparatively low mass). Since all type 1a supernovae emit the same amount of radiation, they are known as standard candles, luminous objects in relation to which scientists can calculate astronomical distances.
This summer, the team is facing the epic digital-age battle of humans versus computers—except they are rooting for the computer, trying to make it more efficient than themselves. They compose synthesized images of outer space based on a program that randomly assigns a value to each pixel. To know the range of values for each pixel, the program draws from real satellite image data. Once the images are simulated, the team tries to pick out the significant supernovae from the “noise,” or the confusing jumble of radiation in the background. They then run the image through a computer program that Brian Connolly created and compare the number of supernovae the computer found to the number they found, hoping that the computer found more.
But more data is about to arrive, and in rapid succession. The SNAP (Supernova Acceleration Probe) satellite, a joint venture of NASA and the U.S. Department of Energy, would take even more resolved pictures of the night sky—and being able to rapidly and effectively distinguish supernovae is necessary for the processing of the data that SNAP collects. Although it is still only a proposed mission, SNAP could launch as early as 2020, so the race for effective supernova-processing software is on.
Today, algorithmic software to detect supernovae exists, but it is still inaccurate and inefficient. The team’s software takes about a day to run a single image—a relatively short amount of time. But, if they can program the software to process the images even faster while maintaining accuracy, it can be much more marketable. And if they continue to have the success they have already found in reprocessing and reanalyzing the algorithms, the team may be achieve its goal of making a more accurate program.
Besides the many hours she spends coding, Becerra enjoys doing “anything musical”; she plays piano, guitar and drums, and has performed as a professional Latin singer in her hometown of Venice, Calif. On campus she sings opera and is a member of the sorority AXL.
Vilsoet spent last semester in the Hamilton program in Paris, where she had plenty of opportunities to pursue her hobbies of going to museums and traveling. Her upcoming senior thesis in physics will most likely pertain to the subject of supernova detection, although she will focus more on the statistical analysis than computation and programming.
Eagan is a member of the cycling team and the president of the Republican club. This summer, he is also simultaneously working on another research project with Professor Connolly comparing algorithms for statistical modeling that will help determine how to create the “best model” for any given situation. As a model becomes more complex, it also becomes more accurate. But to avoid over-complication, different algorithms introduce a penalty for increasing the complexity. The “best model” is one that comes closest to achieving equilibrium between complexity factor and the penalty.
Becerra graduated from New Roads High School in Santa Monica, Calif., Vilsoet from Edgemont High School in Scarsdale, N.Y., and Eagan from Hingham High School in Hingham, Mass.
Posted July 10, 2010