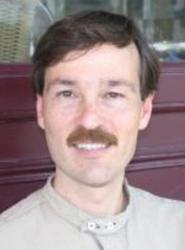
Few people have trouble visually distinguishing a desk chair from a moving car, or the sounds of a crying baby and crashing waves. But could brain activity alone allow researchers to determine what novel stimuli a participant heard or saw? Although the proposition sounds more akin to a science-fiction blockbuster than a scientific possibility, Jack Gallant, of the Gallant Lab at UC Berkeley, has spent decades focused on answering this question.
Gallant visited Hamilton on Sept. 14 to present the Morris lecture, sponsored by the Psychology Department, on the topic of “Mapping, Modeling and Decoding the Human Brain Under Naturalistic Conditions.”
Gallant began with a brief history of fMRI technology, which was developed after WWII, but really gained popularity for research in the 90s. Prior to this breakthrough, most of what was known about neuropsychology was the result of studying lesions (i.e. inferring function in “normal” brains by comparing them to functionally damaged brains). It was during this decade that scientists came to the realization that blood has different magnetic properties when it is oxygenated and deoxygenated, and that this difference was enough to be measured by fMRI.
When these stimuli are presented to research participants in fMRI machines, precise locations of the cortex -- three-dimensional cubes known as ‘voxels’ (akin to three-dimensional pixels) -- are individually measured for increased, decreased or static blood flow. There are about 100,000 voxels, representing 20 billion neurons; however, neurons fire in milliseconds, while fMRI machines can only take measurements every 1-3 seconds. Thus, although fMRI offers unparalleled spatial data, its temporal resolution is severely limited.
Because fMRI machines measure hundreds of thousands of voxels every few seconds over the course of a several hour study, the amount of data produced is unreasonably large. Researchers then use Principal Components Analysis (PCA), to condense the information into 60 principal components that are shared across trials and test subjects.
This proposes a potential limitation, however, in that fMRI does not record neural activity, but rather tracks changes in blood flow. These measurements are collected every few seconds and immediately mapped onto a two-dimensional flattened brain. Gallant and his team found that like stimuli are grouped more closely on the cortex than contrasting words and are organized retinotopically.
Gallant is known for working under naturalistic conditions, that is, with photographs and clips of speech, rather than laboratory created stimuli, such as gabor patches or single phonemes. Gallant and his colleagues’ research on vision eventually became limited by available technology and shifted their to focus to natural language processing.
Gallant chose language because it is organized similar to vision, in that it has small components which form larger pieces and these pieces combine to form a whole. Language and vision are also both hierarchically organized, meaning that if a brain processes an image of a tiger, brain activity will also have been elicited in areas relating to “feline,” “mammal,” “animal,”“organism” and “thing.”
To study natural speech, Gallant turned to NPR’s The Moth and used a computational linguistic model, as opposed to an anthropological model, to see if all subjects share low-dimensional semantic space. Once a model has been established, known as the encoding phase, Gallant and his team present novel stimuli to the participant and measure subsequent blood flow changes. They then take this informati to ‘decode’ what stimuli is being processed. Therefore, “the better the encoding model, the more accurate decoding will be,” Gallant explained.
Unfortunately, fMRI data differs from person to person, as brain shape and size vary. This makes it difficult to pinpoint the same area on two different scans, and poses problems for overlaying data from one brain on another. Previously, this was dealt with by averaging activity across all brains, but this washed out useful data; now, generative modeling allows researchers to characterize underlying brain function by ‘tiling’ functional areas while taking into consideration brains of different shapes and sizes through a process of ‘reverse encoding’ similar to the one for visual stimuli.
This technology has much promise for future research, such as decoding dreams or the mental states of vegetative patients. Gallant himself is excited by the prospect of instantaneous translation decoded purely from mental activity. Although the technology is not close today, Gallant has seen unprecedented leaps and bounds in technological advancements over the past couple of decades and doesn’t expect the trend to slow down.
Posted September 15, 2015